By Shashikant Nishant Sharma
Spatial temporal analysis is an innovative field at the intersection of geography and temporal data analysis, involving the study of how objects or phenomena are organized in space and time. The techniques employed in spatial temporal analysis are crucial for understanding complex patterns and dynamics that vary over both space and time. This field has grown significantly with the advent of big data and advanced computing technologies, leading to its application in diverse areas such as environmental science, urban planning, public health, and more. This article delves into the core techniques of spatial temporal analysis, highlighting their significance and practical applications.
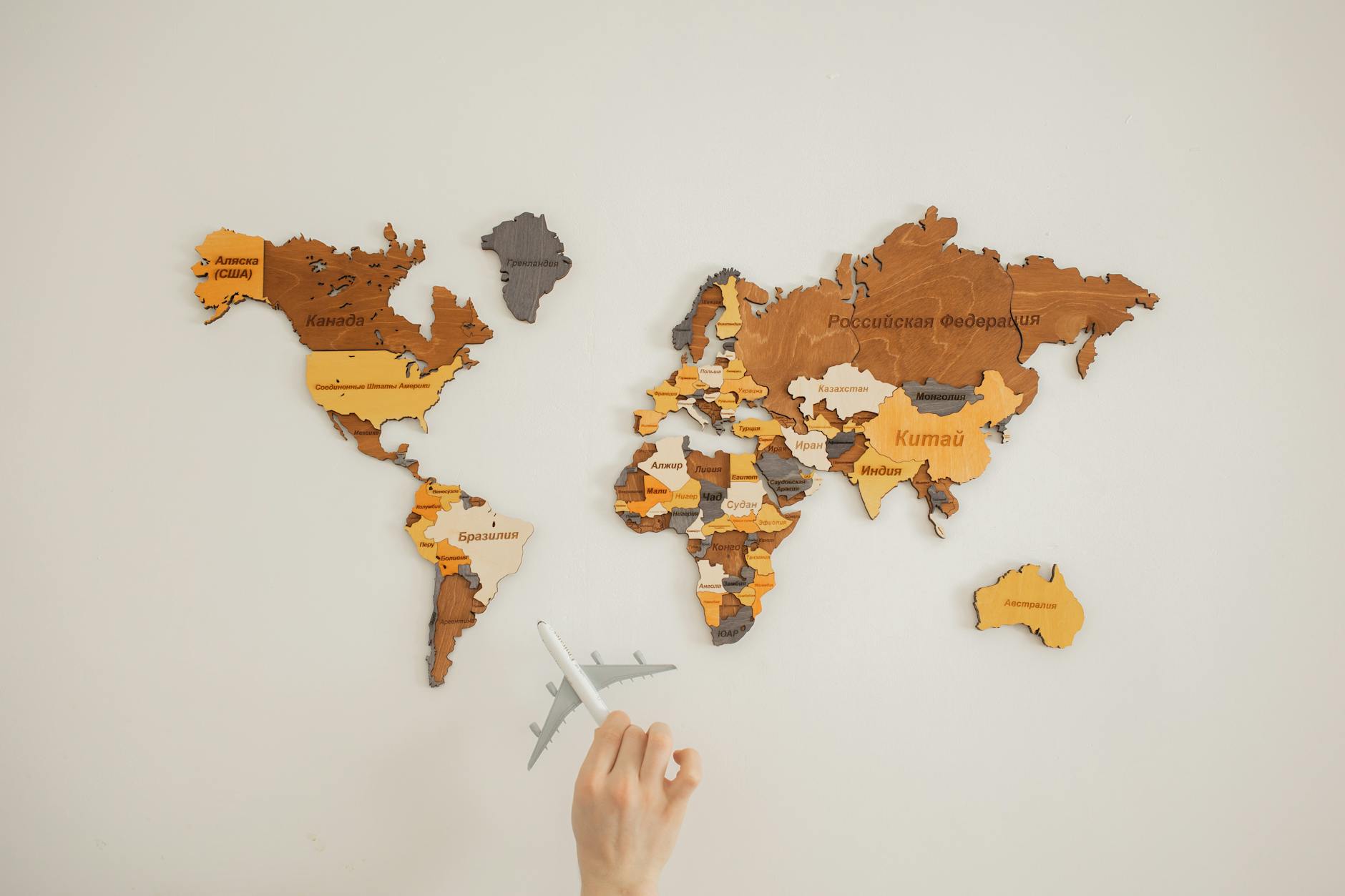
Key Techniques in Spatial Temporal Analysis
1. Time-Series Analysis
This involves statistical techniques that deal with time series data, or data points indexed in time order. In spatial temporal analysis, time-series methods are adapted to analyze changes at specific locations over time, allowing for the prediction of future patterns based on historical data. Techniques such as autoregressive models (AR), moving averages (MA), and more complex models like ARIMA (Autoregressive Integrated Moving Average) are commonly used.
2. Geostatistical Analysis
Geostatistics involves the study and modeling of spatial continuity of geographical phenomena. A key technique in this category is Kriging, an advanced interpolation method that gives predictions for unmeasured locations based on the spatial correlation structures of observed data. Geostatistical models are particularly effective for environmental data like pollution levels and meteorological data.
3. Spatial Autocorrelation
This technique measures the degree to which a set of spatial data may be correlated to itself in space. Tools such as Moran’s I or Geary’s C provide measures of spatial autocorrelation and are essential in detecting patterns like clustering or dispersion, which are important in fields such as epidemiology and crime analysis.
4. Point Pattern Analysis
Point pattern analysis is used to analyze the spatial arrangement of points in a study area, which could represent events, features, or other phenomena. Techniques such as nearest neighbor analysis or Ripley’s K-function help in understanding the distributions and interactions of these points, which is useful in ecology to study the distribution of species or in urban studies for the distribution of features like public amenities.
5. Space-Time Clustering
This technique identifies clusters or hot spots that appear in both space and time, providing insights into how they develop and evolve. Space-time clustering is crucial in public health for tracking disease outbreaks and in law enforcement for identifying crime hot spots. Tools like the Space-Time Scan Statistic are commonly used for this purpose.
6. Remote Sensing and Movement Data Analysis
Modern spatial temporal analysis often incorporates remote sensing data from satellites, drones, or other aircraft, which provide rich datasets over large geographic areas and time periods. Techniques to analyze this data include change detection algorithms, which can track changes in land use, vegetation, water bodies, and more over time. Movement data analysis, including the tracking of animals or human mobility patterns, utilizes similar techniques to understand and predict movement behaviors.
Applications of Spatial Temporal Analysis
- Environmental Monitoring: Understanding changes in climate variables, deforestation, or pollution spread.
- Urban Planning: Analyzing traffic patterns, urban growth, and resource allocation.
- Public Health: Tracking disease spread, determining the effectiveness of interventions, and planning healthcare resources.
- Disaster Management: Monitoring changes in real-time during natural disasters like floods or hurricanes to inform emergency response and recovery efforts.
- Agriculture: Optimizing crop rotation, irrigation scheduling, and pest management through the analysis of temporal changes in crop health and environmental conditions.
Conclusion
Spatial temporal analysis provides a robust framework for making sense of complex data that varies across both space and time. As technology evolves and data availability increases, the techniques and applications of this analysis continue to expand, offering profound insights across multiple domains. Whether through improving city planning, enhancing disease surveillance, or monitoring environmental changes, spatial temporal analysis is a pivotal tool in data-driven decision-making processes. As we move forward, the integration of more sophisticated machine learning models and real-time data streams will likely enhance the depth and breadth of spatial temporal analyses even further, opening new frontiers for research and application.
References
Aubry, N., Guyonnet, R., & Lima, R. (1991). Spatiotemporal analysis of complex signals: theory and applications. Journal of Statistical Physics, 64, 683-739.
Briz-Redón, Á., & Serrano-Aroca, Á. (2020). A spatio-temporal analysis for exploring the effect of temperature on COVID-19 early evolution in Spain. Science of the total environment, 728, 138811.
Cornilleau-Wehrlin, N., Chauveau, P., Louis, S., Meyer, A., Nappa, J. M., Perraut, S., … & STAFF Investigator Team. (1997). The Cluster spatio-temporal analysis of field fluctuations (STAFF) experiment. The Cluster and Phoenix Missions, 107-136.
Dehalwar, K., & Sharma, S. N. (2023). Fundamentals of Research Writing and Uses of Research Methodologies. Edupedia Publications Pvt Ltd.
Gudmundsson, J., & Horton, M. (2017). Spatio-temporal analysis of team sports. ACM Computing Surveys (CSUR), 50(2), 1-34.
Peuquet, D. J., & Duan, N. (1995). An event-based spatiotemporal data model (ESTDM) for temporal analysis of geographical data. International journal of geographical information systems, 9(1), 7-24.
Patel, R. S., Taneja, S., Singh, J., & Sharma, S. N. (2024). Modelling of Surface Runoff using SWMM and GIS for Efficient Storm Water Management. CURRENT SCIENCE, 126(4), 463.
Sharma, S. N., Dehalwar, K., & Singh, J. (2023). Cellular Automata Model for Smart Urban Growth Management.
Sharma, S. N. (2019). Review of most used urban growth models. International Journal of Advanced Research in Engineering and Technology (IJARET), 10(3), 397-405.
Sharma, S. N. (2023). Understanding Citations: A Crucial Element of Academic Writing.
Sharma, S. N. Leveraging GIS for Enhanced Planning Education.
You must be logged in to post a comment.